Financing Infrastructure for a Competitive European AI
Raphaël Doan,
WriterAntoine Levy,
Researcher at the Massachusetts Institute of TechnologyVictor Storchan
Machine Learning engineer10/02/2025
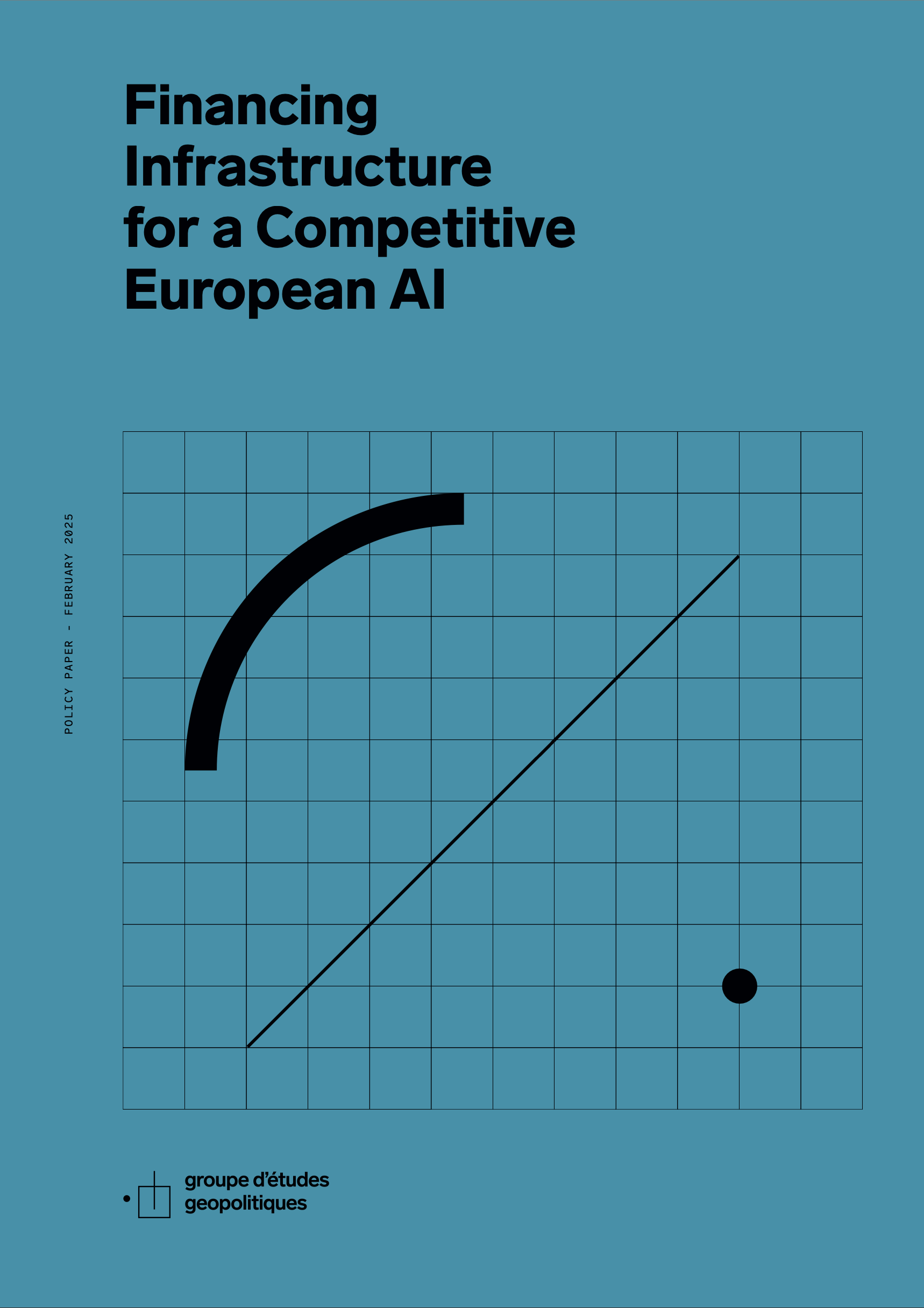
10/02/2025
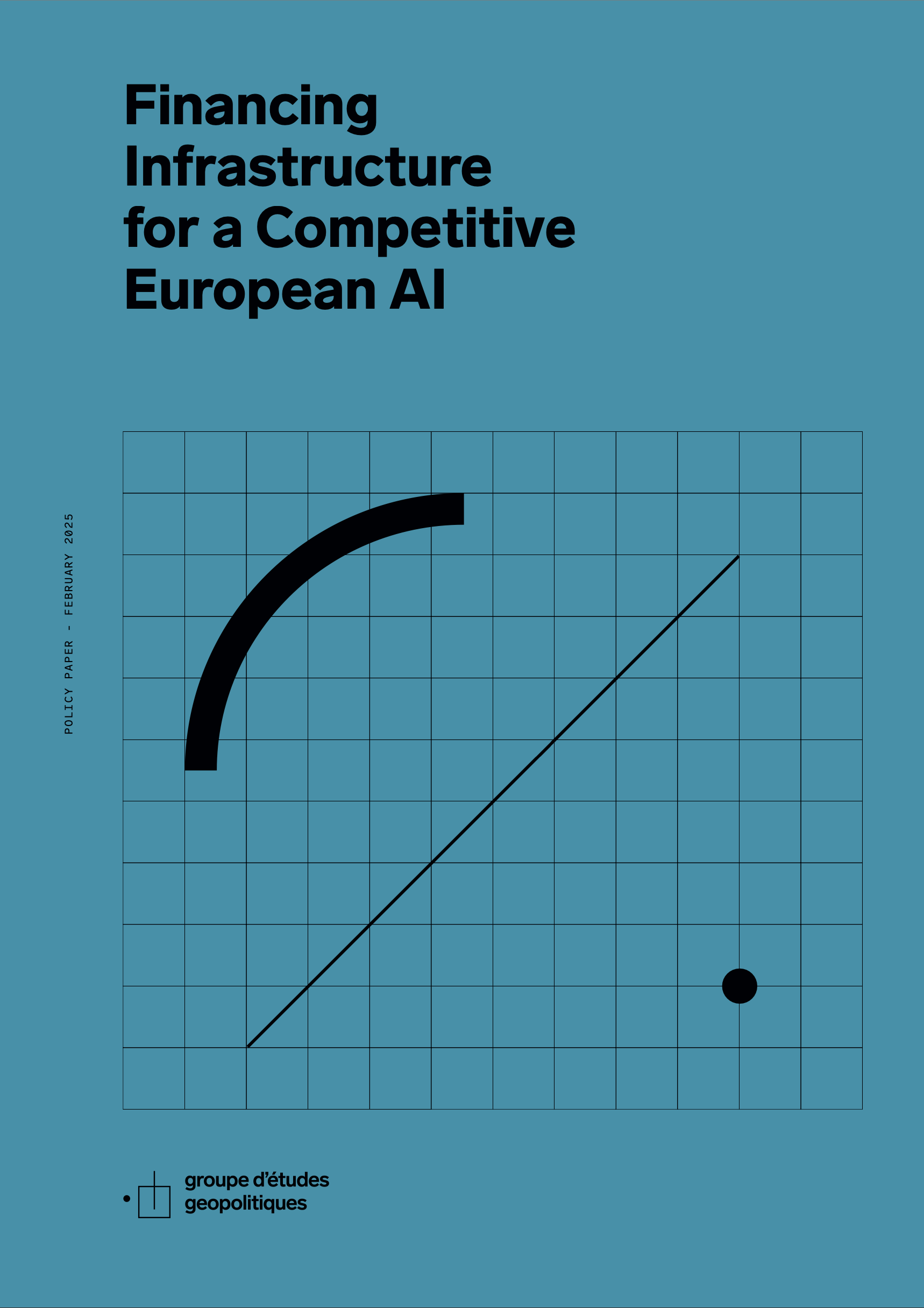
Financing Infrastructure for a Competitive European AI
Executive Summary: Financing Infrastructure for Competitive European AI
Artificial intelligence (AI) is becoming a critical infrastructure for the global economy, comparable to electricity or the internet. By 2030, the majority of cognitive, industrial, and administrative tasks will be augmented or automated by AI, with profound impacts on productivity and economic competitiveness. Without massive, coordinated investment in its infrastructure, Europe risks becoming technologically dependent on the US and China, posing a threat to both its sovereignty and competitiveness. By 2030, economic and technological freedom will have a price: GPUs.
AI infrastructure: the foundation of Europe’s future
- An inevitable economic transformation: By 2030-2035, AI and large language models (LLMs) will become ubiquitous in all sectors (industry, services, healthcare, finance, education). Access to computing power will be as essential as coal was in the 19th century.
- A concerning economic slowdown: Since 2000, European productivity has grown half as fast as in the United States. Without its own infrastructure, Europe will not benefit from the massive productivity gains brought by AI.
- A risk of strategic dependence: At present, 70% of the world’s computing power for AI is held by the United States, 80% of it by American hyperscalers. Europe accounts for just 4% of global capacity, and suffers from industrial energy costs 1.5 to 3 times higher than in the US.
Scalability of AI models: hardware and energy implications
- Schematically, the development of AI models involves two main phases: training (the phase of learning from data) and inference (using the model to generate responses and perform tasks).
- At a given level of performance, i.e. constant capacity, the cost of training an AI model decreases over time (by a factor close to 4 each year) due to gains in hardware performance (improving computing capacity per dollar) and algorithmic efficiency (reducing the number of operations required to train the model). By introducing innovations in model architecture, training methods, and optimizing interconnection speeds between GPUs, DeepSeek has been able to achieve these efficiency gains.
- Model training costs are increasing by a factor of around 2.4 every year. Companies developing AI at the technological cutting edge will not start spending less on training their models. As a result, GAFA CapEx relating to spending on data centers and computing power exceeded 100 billion in 2024, with an increase of over 35% from the previous year.
The size and cost of AI infrastructure in Europe and France
- Today, Europe accounts for just 4% of the global computing power deployed for AI.
- For France, a minimum objective would be to secure computing capacity within its territory dedicated to artificial intelligence that is equivalent to 10% of that of the United States, reflecting its relative weight in US GDP — i.e. around 5-6 GW by 2028.
- If Europe were to set a target of accounting for 16% of global AI computing power by 2030 — in proportion to its weight in the global economy — it would need to increase its AI-dedicated energy power to 20 GW.
- This would put the French target at 200-250 billion euros of investment (i.e. more than twice the amount of 109 billion announced by President Emmanuel Macron on 9 February), and the European target at 500-700 billion.
Financing AI Infrastructure
- Leveraging the EU’s collective borrowing capacity through the reallocation of unused NextGen EU funds and the issuance of new pan-European bonds to finance AI and energy infrastructure.
- Encouraging private investment by adjusting regulatory risk premiums associated with the AI sector and infrastructure financing for insurers and banks, to mobilize additional financial resources.
- Creating dedicated “AI funds”, accessible to European savers without investment limits and eligible for tax-advantaged investment products (similar to France’s PEA), to attract private capital.
- Harmonizing and expanding R&D tax credits across the EU to ensure consistent fiscal treatment of AI infrastructure investments, facilitating seamless cross-border investment in computing clusters.
The need for simplified regulations
- An insufficient legislative framework: Today, it takes at least 5 years to set up a data center in France due to red tape and delays in electrical connectivity.
- Simplification needs to be accelerated: The bill to simplify economic life provides for industrial-scale data centers to qualify as projects of major national interest, enabling certain procedures to be accelerated. Submitted to the French Parliament in April 2024, this text has not yet been voted on by the two assemblies. This approach is mirrored across the Channel in the UK’s “AI Growth zones”, which aim to reduce regulatory barriers to data center construction.
- In France, decarbonized nuclear power can only become an asset for the growth of AI if it is given priority access and the price of its connection is controlled.
In 2012, the Deep Learning revolution gained momentum when the performance of AlexNet, one of the first models trained on two NVIDIA GTX 580 GPUs, was published. Thirteen years later, the infrastructure required to support the development of AI entails unprecedented capital expenditure (CapEx), which neither the private sector nor public powers can afford on their own.
Europe urgently needs to secure the computing power, the necessary energy, and industrial data ecosystem to support ambitious economic and strategic AI goals for the ten years ahead.
For France, on the one hand, this could mean establishing sufficient domestic computing power to support at least five national or European players who are developing foundation models at the technological forefront, while at the same time meeting the minimum usage requirements of its economy’s strategic sectors. In parallel, it is essential to guarantee an adequate energy supply and to build a robust industrial ecosystem around data.
Why invest: AI infrastructure is the foundation of Europe’s future
Whatever the final outcome of the Stargate initiative, the announcement made by OpenAI and its partners at the White House reveals the mindset of American industry and the US federal government: the $500 billion in private investment announced exceeds the Manhattan Project’s $30 billion or the Apollo program’s $250 billion, measured in today’s dollars. This is not just a case of one-upmanship; the medium-term economic value of AI is far greater than the space race of the 1960s. If Europe must make a serious effort, it’s not for the sake of following a trend or chasing after a policy of prestige. It’s a matter of very real power.
The economy of 2030-2035 will be structurally transformed by the omnipresence of large language models (LLM).
The potential applications of AI in healthcare and education are well known, but these are not the only sectors that will be impacted, as it will touch all levels of our businesses, from the most general to the most specific tasks. In this “LLM-ized” economy, the majority of routine cognitive tasks — document analysis, data processing, translation, writing, programming, information searches, micro-decision making — will be augmented or automated by AI. In the manufacturing industry, specialized models will optimize production lines in real time, detect anomalies, and plan maintenance. SMEs will rely on AI assistants to automate their accounting, customer service, or HR processes. In the construction industry, large language models will continuously analyze data from sensors linked to the Internet of Things on building sites to anticipate structural hazards and to sequence work. Architects will generate and test thousands of variations of their plans in function of technical, environmental, and regulatory constraints. Specialized legal models will analyze case law in real time, prepare tailor-made contracts, and detect regulatory inconsistencies. These transformations will even extend to the trades; plumbers and electricians will use assistants to diagnose problems, suggest repairs, or generate a model of a missing part to be 3D printed. LLMs and other core models are on their way to becoming a critical infrastructure, no less essential than electricity or the internet, integrated into most productive processes and economic interactions. This “LLM-ization” of the economy will make access to computing power as critical a factor of production as access to coal was during the Industrial Revolution.
In this respect, Europe’s technological dependence on American infrastructure poses a systemic risk.
Any disruption in the supply of computing capacity — whether due to geopolitical tensions, economic sanctions, or the strategic choices of suppliers — could paralyze whole swathes of the European economy. Critical sectors such as healthcare, energy, or defense would lose their ability to harness AI; in other words they would lose their ability to function properly in ten years’ time. This is not a theoretical vulnerability: U.S. restrictions on chip exports to China and other parts of the world — including parts of Europe — illustrate the reality of this geopolitical lever.
This new risk comes at a time when Europe is already lagging behind the United States in economic terms. Since 2000, real per capita disposable income has grown twice as fast in the U.S. as in the EU. Some 70% of the gap in GDP per capita at purchasing power parity can be explained by lower productivity in Europe. Without control of AI infrastructure — from training to inference — this gap risks becoming a chasm. The massive gains in productivity enabled by AI will primarily benefit those economies with the necessary computing capacity — and will leave out those already known as the “GPU-poor”.
This situation demands a radical paradigm shift in Europe.
The first concerns the allocation of resources. Rather than continuing with the current patchwork of subsidies and investments — which divides its resources — Europe must accept difficult trade-offs and concentrate its investments. The costs involved are staggering, and will inevitably mean giving up other initiatives and areas of action. This is a gamble we cannot afford to pass up. We should point out that this does not only concern governments: private French and European fortunes have the means to put the Union on the global AI map, provided, once again, that they do so in a focused way.
On the data aspect, Europe needs to move beyond a vision which is solely focused on the protection of personal data to embrace the challenge of collecting and making accessible training data. Public agencies, which have a treasure trove of data in health, education, and energy, must set an example by making it accessible for use in AI; this is not incompatible with the confidentiality of purely personal data and would open the door to real advances.
By aiming to cover the essential inference needs of our future economy, we are proposing a clear and understandable objective. Of course, we can argue about the calculation and the underlying assumptions. But it is, in our view, the correct criteria to keep in mind; this is not a question of doing research for research’s sake, nor of deciding in advance which technological choices should be left up to businesses. It’s about anticipating, by any means necessary, our future computational independence.
Two hundred years ago, the Industrial Revolution needed a century to reconfigure the anthropological balance of Europe and the world. What we are seeing in the possibilities offered by AI promises upheaval on a comparable scale, but in a much shorter time frame. A Europe that is dependent on foreign infrastructure would lose all ability to shape its own economic and social destiny. Automating any cognitive tasks that can be automated is too important a challenge for us to leave to anyone but ourselves. By 2030, freedom will have a tangible price: processors.
Scalability of AI models: hardware and energy considerations
Training and inference
The development of AI models can be divided into two main phases: training, which consists of learning from data, and inference, which corresponds to its practical application to generate responses and carry out tasks in real time.
For the most advanced AI models, training relies on large-scale clusters of graphics processing units (GPUs) that are interconnected in specialized data centers. When developing a model, it is essential to carry out small and medium-scale exploratory training runs to test and validate architectural choices, training optimizations, or data allocation strategies before launching a final, large-scale training run. These intermediate phases must be factored into the model’s total cost when estimating the computing capacity required to operate at the technological cutting edge. Inference, by contrast, can be run on less powerful GPU clusters or “edge” devices — the process of running AI models directly on local devices (such as smartphones, IoT devices, or in cars) — depending on usage needs. Unlike data centers dedicated to training, those specialized in inference are located close to where they will be used in order to reduce latency between users and servers.
The scaling laws for AI
The empirical scaling laws of AI indicate that, all other things being equal — notably the quality of training data — for optimal training, computing power must be divided equally between increasing model size and increasing data quantity. So, while budgets for training models continue to grow, the size of both datasets and models are increasing in proportion. Globally, the cost of training the most advanced models has increased by a factor of 2 or 3 over the last eight years 1 , reaching tens to hundreds of millions of dollars. For example, OpenAI’s GPT4 model trained in 2022 (around 2e25 FLOPs) used a cluster of 20K A100 GPUs and consumed 15-20 MW of power. Meta’s Llama 3 model, trained in early 2024 (3.8e25 FLOPs), used 16K H100 GPUs from a cluster of 24K GPUs, and Llama 4 is expected to use more than 100K H100 GPUs 2 .
Similarly, the emergence of reasoning models (DeepSeek-R1, O1-mini, O3-mini etc.) has shown that it is possible to scale up along a second dimension: at inference, performance is also an increasing function of the amount of computation allocated for the model to run and test several lines of reasoning.
Algorithmic and hardware efficiency
As a result of research and technological innovation, we can observe a dual dynamic. Algorithmic efficiency — model architectures, optimization and training methods — is advancing towards reducing the amount of computation required to achieve a given performance. At the same time, GPU performance, for a given price, is increasing — doubling every two years between 2006 and 2021 3 . As a result, estimates 4 show that the level of computation required — and therefore the cost — to achieve a given level of performance falls by around half every 8 months. Other observations suggest that, for a given level of performance, the cost of a model will be divided by 4 each year thanks to technological advances. In other words, if it costs $100 million to train a model today, the cost will fall to $25 million a year later, then to $6 million in two years’ time, and so on.
The most striking recent example of algorithmic efficiency gains is DeepSeek.
The Chinese company achieved greater efficiency in particular through innovation including in the model architecture (never before seen sparsity factor, MLA, GRPO, etc.) or by rewriting in assembly language (PTX) the communications between GPUs and the nodes of their clusters in order to overcome the interconnection speed limitations of H800 GPUs. These innovations allowed DeepSeek to make better use of its resources for both training and inference. This does not, however, mean the end of scaling laws in AI.
Companies developing AI at the cutting edge of technology are not going to start spending less on training their models. For a given model capacity, increased algorithmic and hardware efficiency means a reduction in the training cost to develop the model, as well as a reduction in inference costs — decreasing by a factor of 10 every year for the past 3 years 5 . However, when operating at the technological frontier, AI labs are finding new dimensions to scale up 6 (pretraining, RL, computation time for inference, etc.), requiring unprecedented computing capacity. This is reflected in the capex of the major US cloud providers: in 2024, hyperscalers spent a total of over $100 billion on AI infrastructure 7 . The price of a gigawatt data center equipped with the latest NVIDIA GB300 chips is estimated at $40-50 billion. As a point of reference, DeepSeek’s cost in computing power is estimated at 100 million 8 per year, and certainly around $500 million since the company began operations.
International comparisons and the state of AI infrastructure strategies
A European AI infrastructure and insufficient funding
Today, Europe accounts for just 4-5% of the world’s computing power deployed for AI 9 .
European cloud companies account for a market share of less than 5% 10 . Looking at the world’s leading AI startups, 61% of global funding goes to US companies, 17% to Chinese companies, and only 6% to EU companies 11 . As far as data centers are concerned, Europe hosts a total of 18% of the world’s data center capacity, of which less than 5% is owned by European companies, compared with 37% for the US 12 , a comparable economy. European industrial tariffs (0.18 USD/kWh on average) are up to three times higher than in the US, making AI infrastructure more expensive: some estimates calculate the cost of setting up data centers in Europe to be 1.5 to 2 times higher than in the US 13 14 . As such, in June 2024, the French company Mistral AI warned of the lack of computing capacity for training AI models on European soil 15 .
European strategy for AI infrastructure
The European Commission has announced an AI Factories plan built around member states’ supercomputer projects, which are primarily dedicated to public research.
This investment of 1.5 billion euros is part of the Digital Europe program, which is funding AI — data infrastructure, evaluation and dissemination of AI in the economy — with up to 2.1 billion euros for the period 2021-2027 and 2.2 billion for upgrading or building supercomputers 16 .
International strategies for AI infrastructure
National governments are stepping up their efforts to attract private investment to fund strategic AI infrastructure — a key lever in geopolitical and economic dynamics.
These initiatives are part of a growing competition for technological leadership.
In the United States, even if there is some skepticism surrounding the $500 billion in investment and the execution of the Stargate project, it does not negate the fundamental need for Europe to develop its AI infrastructure at scale — the bursting of the dot-com bubble didn’t hinder the emergence of cloud technology players.
In the UK, the AI Opportunities Action Plan introduces “AI growth zones”, which fast-track approvals for data center construction and facilitate access to the energy grid.
The Bank of China has announced a 1 trillion yuan ($140 billion) financing plan to support AI companies engaged in foundational research and the industrialization of AI 17 . A plan to build eight computing centers and ten national data centers was also approved 18 .
Global demand and supply
TSMC forecasts that demand for AI-dedicated servers will grow by 50% per year over the next five years 19 . On the production side, estimates predict annual growth of 35%-60% in the volume of GPUs available 20 .
The size and cost of AI infrastructure in Europe and France
In Europe
Global demand in critical power for data center computing will grow from 49 gigawatts (GW) (of which 5 GW is AI) in 2023 to 130 GW by 2030 21 , of which around 40 GW will be consumed by AI.
The situation in the U.S. is as follows: in 2023, the total power of American data centers is estimated at 23 GW, representing around 5% of the country’s total electrical capacity — including 3.3 GW specifically allocated to AI. Based on demand for specialized graphics cards, projections for 2028 indicate that total U.S. data center power will reach 83 GW, including 56 GW dedicated to AI. This would place the US’ share at around 70% of the world’s power dedicated to AI — 80% of which would be held by US hyperscalers.
Today, Europe accounts for 4 to 5% of the AI computing capacity deployed worldwide, or 0.25 GW5 22 . If Europe were to set a goal for itself to account for 16% of global AI computing power by 2030 — proportionate to its weight in the global economy — it would need to increase its energy power dedicated to AI to 20 GW. We find a similar order of magnitude if Europe were to set the target of catching up with the US in terms of the share of electrical power allocated to AI in data centers by 2030 (installation of 17 GW 23 ).
In France
For France, a minimum objective would be to secure a computing capacity dedicated to artificial intelligence within its borders equivalent to 10% of that of the United States, reflecting its relative weight in American GDP — i.e. around 5-6 GW by 2028.
Depending on the distribution of power between inference and model training at the technological forefront — about 10^25 FLOPS today and 10^26 FLOPS by 2027 24 — this would enable France to support the computing power of 3 to 5 world-class players on its soil. In 2024, 40% of Nvidia’s data center revenue was related to inference. Google indicates that between 2019 and 2021, inference accounted for around 60% of total AI computing in its business 25 .
Cost
As previously mentioned, the cost of installing 1 GW of next-generation Nvidia GB300 GPUs is estimated at 40 or 50 billion euros 26 . For the current Hopper generation (H100), 1 GW of installation could represent a cost of 15 to 23 billion depending on hardware depreciation and market adjustment factors.
In other words, this would put the French target at 250-300 billion euros of investment, and the European target at 500-700 billion.
Which financing
To fund the colossal investments required, both private and public funds must be mobilized. The collective borrowing capacity of EU member states, which is currently under-utilized, could be made available, particularly by redirecting unused or uncommitted funds from the NextGen EU plan, as well as by issuing new pan-European bonds dedicated to financing cluster and power generation infrastructure. On the private financing side, the modulation of regulatory risk premiums associated with the AI sector and infrastructure funding for insurers (Solvency 2 directive) and banks (Basel III agreements) is a potential avenue for mobilizing capital from retirement savings and life insurance in particular. More broadly, the possibility could be studied of raising dedicated “AI funds”, which would be accessible to European savers, with no limit on the amount, and would be eligible for tax-exempt investment products (like the PEA in France).
From a business point of view, the generalization and systemic harmonization of innovation and research tax credits throughout the Union would ensure fiscal continuity in the treatment of cluster investments between countries in the zone. The tax treatment of expenditure on data acquisition, maintenance and storage could also be adjusted to allow accelerated depreciation of these investments, given the need for the most advanced countries to catch up quickly in this area.
The need for simplified regulations
Increased funding is a necessary but insufficient prerequisite for computing power. The development of infrastructure on European and French soil is hampered by numerous administrative procedures, legal appeals, and delays in the electrical connection of data centers. In all, it takes at least 5 years to set up a data center in France.
In order to reduce these delays, and in line with the recommendations of the Artificial Intelligence Commission, the proposed law for the simplification of economic life provides for industrial-scale data centers to qualify as projects of major national interest, allowing certain procedures to be fast-tracked. Submitted to the French Parliament in April 2024, the bill has not yet been voted on by the two houses of parliament. Across the Channel, this move is mirrored in the UK’s “AI Growth zones”, which aim to reduce regulatory barriers to data center construction.
Beyond the imperative need to complete this normative and procedural simplification process, it will also be necessary to make it easier for RTE — and its European national equivalents — to connect data infrastructures to the power grid. In France, decarbonized nuclear power can only become an asset for developing AI if access to it is prioritized and the cost of connection controlled.
Notes
- Ben Cottier, Robi Rahman, Loredana Fattorini, Nestor Maslej, and David Owen. ‘The rising costs of training frontier AI models’. ArXiv [cs.CY], 2024. arXiv. https://arxiv.org/abs/2405.21015.
- Jowi Morales, «Meta is using more than 100,000 Nvidia H100 AI GPUs to train Llama-4 — Mark Zuckerberg says that Llama 4 is being trained on a cluster “bigger than anything that I’ve seen”», Tom’s Hardware, 31 October 2024.
- Konstantin Pilz, Lennart Heim, Nicholas Brown, «Increased Compute Efficiency and the Diffusion of AI Capabilities», 13 February 2024.
- Anson Ho, Tamay Besiroglu, Ege Erdil, David Owen, Robi Rahman, Zifan Carl Guo, David Atkinson, Neil Thompson, and Jaime Sevilla. ‘Algorithmic progress in language models’. ArXiv [cs.CL], 2024. arXiv. https://arxiv.org/abs/2403.05812.
- Guido Appenzeller, «Welcome to LLMflation – LLM inference cost is going down fast», Andreessen Horowitz, 12 November 2024.
- Nikhil Sardana, Jacob Portes, Sasha Doubov, Jonathan Frankle, «Beyond Chinchilla-Optimal: Accounting for Inference in Language Model Scaling Laws», 31 December 2023.
- Jérôme Marin, «En 2024, Microsoft, Amazon, Google et Meta ont dépensé 100 milliards de dollars dans leurs infrastructures d’IA», L’Usine Digitale, 19 December 2024.
- Nathan Lambert, «DeepSeek V3 and the actual cost of training frontier AI models», Interconnects, 6 January 2025.
- Dylan Patel, Daniel Nishball and Jeremie Eliahou Ontiveros, «AI Datacenter Energy Dilemma – Race for AI Datacenter Space», SemiAnalysis, 13 March 2024.
- Alexander Sukharevsky, Eric Hazan, Sven Smit, Marc-Antoine de la Chevasnerie, Marc de Jong, Solveigh Hieronimus, Jan Mischke, and Guillaume Dagorret, «Time to place our bets: Europe’s AI opportunity», McKinsey, 1 October 2024.
- Mario Draghi, «The Future of European Competitiveness», European Commission, September 2024.
- Alexander Sukharevsky, Eric Hazan, Sven Smit, Marc-Antoine de la Chevasnerie, Marc de Jong, Solveigh Hieronimus, Jan Mischke, and Guillaume Dagorret, «Time to place our bets: Europe’s AI opportunity», McKinsey, 1 October 2024.
- Dylan Patel, Daniel Nishball and Jeremie Eliahou Ontiveros, «AI Datacenter Energy Dilemma – Race for AI Datacenter Space», SemiAnalysis, 13 March 2024.
- Alexander Sukharevsky, Eric Hazan, Sven Smit, Marc-Antoine de la Chevasnerie, Marc de Jong, Solveigh Hieronimus, Jan Mischke, and Guillaume Dagorret, «Time to place our bets: Europe’s AI opportunity», McKinsey, 1 October 2024.
- Cynthia Kroet, «Mistral AI warns of lack of data centres and training capacity in Europe», Euronews, 14 June 2024.
- Digital Europe Programme (DIGITAL) | EU Funding & Tenders Portal, European Commission.
- Sharveya Parasnis, «Bank of China Announces Investments Worth 1 Trillion Yuan to Develop AI Industry» Medianama, 28 January 2025.
- «China approves mega project for greater computing power, digital future», Popular Republic of China, 18 February 2022.
- «Q1 2024 Taiwan Semiconductor Manufacturing Co Ltd Earnings Call», 18 April 2024.
- Jaime Sevilla et al. (2024), «Can AI Scaling Continue Through 2030?», Epoch AI.
- Tim Fist and Arnab Datta, «How to Build the Future of AI in the United States», IFP, 23 October 2024.
- This represents around 3% of the total 10 GW of electrical power installed in European data centers.
- Total installed electrical power demand for data centers in Europe is estimated at 35 GW by 2030.
- Pilz, Konstantin F., Yusuf Mahmood, and Lennart Heim, AI’s Power Requirements Under Exponential Growth: Extrapolating AI Data Center Power Demand and Assessing Its Potential Impact on U.S. Competitiveness. Santa Monica, CA: RAND Corporation, 2025.
- Tim Fist and Arnab Datta, «How to Build the Future of AI in the United States», IFP, 23 October 2024.
- «NVIDIA GB300 “Blackwell Ultra” Will Feature 288 GB HBM3E Memory, 1400 W TDP», 23 December 2024.
citer l'article
Raphaël Doan, Antoine Levy, Victor Storchan, Financing Infrastructure for a Competitive European AI, Feb 2025,